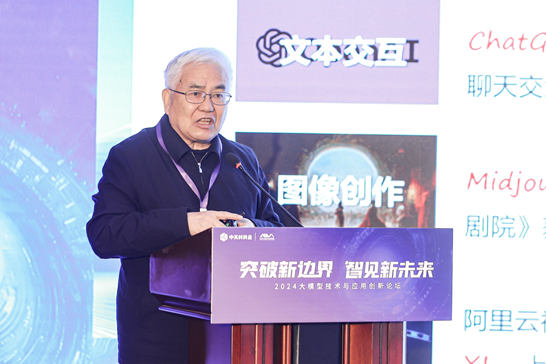
Text丨Zhou Wenmeng of Sina Technology
In the process of training big models of artificial intelligence, purchasing supercomputing services or memories is also becoming a new way to effectively relieve computing power anxiety.
Recently, Zheng Weimin, an academician of the Chinese Academy of Engineering and a professor of computer science at Tsinghua University, pointed out in a communication with Sina Technology, “In the past, training a big model required several billion dollars, but if big model training was carried out using supercomputing, it would only cost one-sixth of Nvidia's.”
Furthermore, Zheng Weimin also pointed out a new trend in the development of AI reasoning — “save-to-save conversion.” Using the Mooncake technology framework jointly developed by Tsinghua University and the AI unicorn company Dark Side of the Moon as an example, he introduced the principle that the technology is based on the “save-to-save conversion” idea to help the Dark Side of the Moon Kimi smart assistant relieve tight computing power requirements, thus avoiding server downtime.
“Move big model training to supercomputing, the price is only 1/6 of Nvidia”
Zheng Weimin saw that after being quickly caught up by various technology companies around the world after the release of ChatGPT, this year's big model has two characteristics: first, the basic big model has entered a multi-modal state, not only text, but also images, videos, etc.; second, it has actually been used. The big model is actually being integrated with various industries, such as big model+finance, big model+medical, big model+intelligent manufacturing, etc.
“The big model is really closely integrated with the GDP of the national economy and people's living standards. I always think that our standard in the basic big model is still a little worse than that of the US, but with the 'big model+' thing, we still have hope of surpassing the US.” Zheng Weimin said.
However, in the actual application process of a large model, a large amount of computing power resources is required in the entire life cycle, which includes the five steps of data acquisition, data preprocessing, model training, model fine-tuning, and model inference. How to obtain more efficient and reliable AI big model computing power resources at a lower cost has become a question that every company is thinking about.
Due to difficulties in obtaining high-end chips from overseas, one of the main solutions developed domestically to meet the massive computing power requirements brought about by AI large model training is to meet the massive computing power requirements of one's own large-scale model product training by building kilocalories and 10,000 card clusters, purchasing chips from multiple manufacturers to conduct joint training with heterogeneous cards through massive chip semiconductor stacking. However, according to Zheng Weimin, although this method can solve the problem of scarce computing power, it also has some drawbacks.
First, for building a domestic 10,000 card system, it is certainly important to build it, but it is very difficult to use it well. Zheng Weimin said with his own personal experience of doing high-performance computing: “Build a 2,000 card system, with 1,000 Nvidia chips and another 1000 from other manufacturers. The system was built and operated, but in the end, it was discovered that these chips had different performance. Some had smaller skills, some had larger skills, and one task was divided into 2,000 parts. In addition, 1000 tasks were divided into smaller tasks. If it was dynamic, it was directly divided into 2,000 copies for processing and performance. Very low.”
Zheng Weimin pointed out that during the construction of large-scale computing power clusters, there was a wooden barrel effect. Some computing cards are powerful, while others are weak. Just as how much water is contained in the entire barrel is ultimately determined by shortcomings, it is useless no matter how long the board is. “So when 1,000 old GPUs are combined with 1,000 new GPUs, the performance is a little lower than that of 2,000 old GPUs, and the cost of building a large-scale computing power cluster is also quite high.”
According to Zheng Weimin, carrying out large-scale joint training with heterogeneous cards is very difficult and uneconomical. If offsite cards are involved, it will be even more difficult. The data is transmitted from Beijing to Guizhou, and Guizhou produces results and then sent to Shanghai. The time costs involved in this are extremely high. “People with less money don't need to do it; people with more money can try it.”
Zheng Weimin suggested that companies try using supercomputing to train large AI models. “Our country has a 1.4 billion supercomputing system. The money is paid by the state, and some machines have a bit of surplus. Because domestic supercomputing machines are cheap, unlike Nvidia, which has to make money if they want to recover the cost of the machine, it's enough for everyone to increase their model training and do it on Qingdao Shenwei Supercomputing.” Zheng Weimin said.
“Converting to savings can effectively reduce AI inference costs”
In fact, in the actual application process of a large model, during the entire life cycle involving the five steps of data acquisition, data preprocessing, model training, model fine-tuning, and model inference, a large amount of computing power resources are required, while also having a large amount of storage resources to store massive calculation results. Especially in the model reasoning process, how to store much more, communicate quickly, and is cost-effective has become a question that the entire industry is thinking about together.
Earlier, Zheng Weimin publicly mentioned, “AI storage is the key foundation of the big model of artificial intelligence. The storage system exists in every part of the life cycle of the big model and is the key foundation for the big model. Through forced calculation and store-to-store conversion, advanced AI storage can improve the availability of training clusters, reduce inference costs, and enhance the user experience.”
In communication with Sina Technology, Zheng Weimin shared the basic principle of “deposit-to-deposit conversion.” He pointed out, “Whether it's training or inference, large models require a lot of computing power, and also require a lot of storage to store the massive parameters trained by the big model, as well as some process data generated during the inference process.” However, if more and more data needs to be stored throughout the training or inference process, this will lead to a shortage of memory resources, which will eventually become a “burden” for improving the performance of large models.
According to Zheng Weimin, in order to solve the above problems, Tsinghua University has thought of two solutions: First, in the inference process, the main inference card is currently mainly used, and the host CPU and host memory are not used. Therefore, it is possible to find ways to use the memory on the host computer during the inference process, which improves memory utilization. While improving performance, it also saves the capital costs of continuously purchasing inference cards; second, by storing the data generated during the inference process, which is directly used by users when similar problems are encountered later, it can be directly used and eliminated The reasoning process each time a similar problem is encountered, improving efficiency and saving resources.
Using the Mooncake technology framework jointly developed by Tsinghua University and Dark Side of the Moon as an example, Zheng Weimin pointed out, “By extracting and storing public content of conversations between different users and Kimi, this not only reduces the process of having to be regenerated every time a user asks a question, saving many computing power cards, but also reducing problems such as 'access delay' or 'downtime' caused by Kimi's excessive visits.”